When faced with counting a large number of objects on many images after first thinking of graduate students, one should thing of SAMPLING. That science is well-established and known by many but even more who should know better never think of it.
Below is a brief outline of two of many approaches that involve sampling. The first method uses just simple random sampling and the human eye-brain system. The second example combines the human eye-brain system and the ingenious methods described by the other answers to the original question.
Simple random sampling without replacement
Divide the image into a grid of non-overlapping cells. Randomly select $n$ of those without replacement. The estimate of the total number of objects is just the mean count times the total number of cells. A measure of precision (also essential but many times ignored or forgotten) can also be estimated. Here is some Mathematica code to do that:
(* Figure out the boundaries for nGroups in each direction *)
split[nPixels_, nGroups_] := Module[{k, nkPlus1, nk, xUpper, xLower},
k = Floor[nPixels/nGroups];
nkPlus1 = nPixels - k nGroups;
nk = nGroups - nkPlus1;
x = If[nkPlus1 == 0, ConstantArray[k, nk], Join[ConstantArray[k, nk], ConstantArray[k + 1, nkPlus1]]];
xUpper = Accumulate[x];
xLower = Join[{1}, Most[xUpper + 1]];
{xLower, xUpper}
]
(* Given image, split into a nGroups x nGroups grid of cells
and sample n of those cells without replacement *)
sample[img_, nGroups_, n_] := Module[{},
(* Get random sample of cells without replacement *)
col = split[ImageDimensions[img][[1]], nGroups];
row = split[ImageDimensions[img][[2]], nGroups];
indices = Flatten[Table[{i, j}, {i, nGroups}, {j, nGroups}], 1];
samples = RandomSample[indices, n];
(* Now ask the user to count objects in each cell *)
counts = ConstantArray[0, n];
Do[imgSample =
ImageTake[img, row[[All, samples[[i, 1]]]],
col[[All, samples[[i, 2]]]]];
counts[[i]] = Input[imgSample], {i, n}];
(* Return estimate of total number of objects and an associated standard error *)
{Mean[counts] nGroups^2,
Sqrt[1 - n/nGroups^2] StandardDeviation[counts] nGroups^2/Sqrt[n]} // N]
(* Example *)
(* Divide image into a 5x5 grid of sample units and take a sample size of 6 *)
sample[img, 10, 6]
(* {333.3333333333333`,73.69607256232254`} *)
One will be asked to make 6 counts by eye with dialog boxes looking like the following:
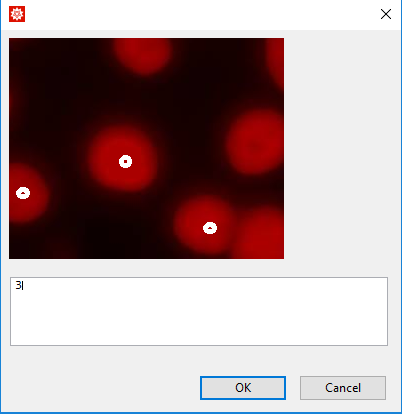
Here I've marked the objects counted by eye. (What counting rule was used? Count all objects that are totally self-contained within the cell. Also count the objects that intersect the left and bottom border of the cell but excluding any objects that intersect with the right-hand lower corner of the cell. This is an attempt to reduce boundary issues that affect the count. The sides used and corner excluded could be randomized.)
So the estimate is 333 objects with a standard error of around 74. Not great but one can do much better with the next example.
Ratio estimation
Ratio estimation is a standard statistical technique where one has the ability to make a reasonable (but not necessarily perfect) count for all of the grid cells. Then the "exact" measurement (i.e., human eye/brain system) only needs to be taken on a sample of the grid cells. If the estimate of the number of objects is $X$ from one of the ingenious methods using the Mathematica functions and $\bar{x}$ and $\bar{y}$ are the mean counts of the "reasonable" and "exact" sampled cells, the estimate of the total number of objects in the image is just
$$\bar{X} {\bar{y} \over \bar{x}}$$
An estimate of precision is also easily calculated. If the use of Mathematica functions results in counts proportional to the true count, then the precision will be much, much better than simple random sampling. (I'll add details when I get more time.)