The code below replaces pile
with a function avalanche
that works a bit bit differently! Instead of calling itself, it outputs a list of things that we then want to recursively call avalanche
on again. When the list is empty (there are no more things to compute), we stop. To implement the recursion, we use NestWhile[f, init, testfunction]
.
the avalanche
function
Here f
isn't precisely avalanche
, but let's first detail exactly what avalanche
does:
On an argument {x, y}
, it checks if sandpile[[x,y]]
is over capacity, and if so, reduces it by four: sandpile[[x,y]] -= 4
.
Forms the neighbors of {x,y}
by, via makeneighbors
*, giving us a list of neighbors {x,y} + r
for every r
in neighbors
(except those that wind up the grid). (See makeneighbors
at the bottom for that function's implementation.)
Replaces each of these elements (at level 1 only; that's the {1}
that is the last argument to Replace
) with itself if iterating the sandpile at that spot is over capacity, and delete it (replace it with Nothing
) otherwise. There's maybe a better way of doing this, but this was the first (well, second; the first idea didn't work, actually!) that came to mind. This is weird, because it actually performs the iteration of a spot in sandpile
by executing the test. So, this is probably Bad Code, but hey, kinda cool that you can do that!
So, the overall behavior of avalanche
is: take in a point {x,y}
, perform updating of sandpile
for it and its neighbors, return the list of neighbors that are now over capacity.
building the function for NestWhile
out of avalanche
We now want a function f
in NestWhile
that takes in a list of such over-capacity neighbors, does avalanche
on each of them, and smushes together all the resulting lists of over-capacity positions into one list of positions, deleting duplicates.
#
is our stand-in for the list of neighbors as we build this anonymous function. To do avalanche on each of the elements of the list, we can do avalanche /@ #
. The result is a list of lists of positions.
Union
can then do the, er, set union, as you might expect, but it takes in arguments as Union[list1, list 2, ...]
. We have {list1, list2, ...}
. So we need to replace the head of this (which is List
: {a, b, ...}
is just List[la, b, ...]
) with Union
. We do that by Union @@
, giving us the overall function (Union @@ (avalanche /@ #)) &
that we use in NestWhile
.
Note that g /@ {}
is {}
, and Union @@ {}
is Union[]
is {}
, so eventually, when no points are over capacity, we will have {}
as output, and we want to stop the loop. So our testfunction
ought to check if the output is {}
, i.e. # == {} &
.
We want to start the NestWhile
on just one point—or, more precisely, the list containing one point, since we always expect the input/output in NestWhile
to be a list of points. Hence {{x,y}}
.
So, that's that for the actual function behavior!
visualization
Here's how the visualization works: we decide we want to pause for a tenth of a second each time we go through the NestWhile
loop at least twice. (The way the current code is, we go through once every time. It might help to change that by moving the check for sandpile capacity via sandpile[[x,y]]
outside the function avalanche
and outside the whole loop, so we never enter the loop if we don't need to. But I didn't do that here to keep it close to the original code.)
One way we can do this is by having a virtual switch p
which is 1
before we've executed the function in NestWhile
, but gets flipped off once we go throuh the NestWhile
loop, after we've checked. So we make p = 1
in every Do
iteration, and before executing our function f
, we check if p
has been flipped to 0
yet. If p
has been flipped, we pause for 0.1
seconds. If not, it's the first time through, and we don't pause, and instead just flip p
to 0
. After all this we execute our usual function. So our function has gone from f
as above to If[p == 0, Pause[0.1], p = 0]; f
. Here, though, we also want to check that we've passed iteration 15000
, since the first avalanches are very small and not very interesting to watch. So we add the check m > 15000
to get If[p == 0 && m > 15000, Pause[0.1], p = 1]; f
(Note: I just realized this isn't the Do
loop variable! The variable n
(given {n,nmax}
) already keeps track of the iteration of the Do
loop for us. So we could have just used n
instead of m
.)
For colors, we just set ColorFunction
in ArrayPlot
to something that's gray below or at 4, and magenta above. We also need to turn off ColorFunctionScaling
otherwise all values will be scaled to lie between 0 and 1 before we apply the color function, but we want our color function to take in raw values.
*makeneighbors
Named this function for convenience! Taking in a point {x,y}
, it maps the function ({x,y} + #) &
over the list of neighbors
via /@
.
It then selects from this list the ones that have each component lying in the appropriate bounds, by applying AllTrue[#, 1 <= # <= L &] &
to test them. (Note that the first #
will be filled by the neighboring point itself as a list of coordinates, whereas the second represents a component of that list of coordinates.) This gives us a list containing {x,y} + r
for every element r
of neighbors
, without the ones that lie outside the grid.
L = 100;
neighbors = {{0, 1}, {1, 0}, {0, -1}, {-1, 0}};
(*Make the neighbors that satisfy the bounding constraints in all of
their (2) components*)
makeneighbors[{x_, y_}, L_] :=
Select[({x, y} + #) & /@ neighbors, AllTrue[#, (1 <= # <= L &)] &];
(*This replaces the pile function. We want avalanche to 1) have the side-effect of
modifying all the neighbor cells and 2) return a list of those
neighbors which are now over capacity. When we use this function,
we'll want to map it back over all of its outputs via a NestWhile.
(Note that we could move the first check of capacity out of the
function, as it's kind of redundant.) *)
avalanche[{x_,
y_}] :=
If[
sandpile[[x, y]] > 4,
sandpile[[x, y]] -= 4;
(*generate the neighbors,
then keep the ones that became over capacity after incrementing
them; delete the ones that don't become over capacity. Note that the
increment happens inside the test, which is kind of weird! (Also, ++x is
the one that returns the new value of x (as opposed to x++), so we really are
testing the new value.) So, the function avalanche returns a list of newly "hot"
or "precarious" points.*)
Replace[makeneighbors[{x, y}, L], {a_, b_} :>
If[++(sandpile[[a, b]]) > 4, {a, b}, Nothing], {1}],
(*If it wasn't over capacity, just return the empty list, as there are no "hot" points.*)
{}
];
nmax = 100000;
m = 0;
sandpile = RandomInteger[{0, 3}, {L, L}];
Monitor[Do[{x, y} = RandomInteger[{1, L}, {2}];
(*indicate that we're on a new iteration,
and haven't triggered any avalanches yet (for visualization purposes)*)
p = 1;
(*add a grain to our random site*)
sandpile[[x, y]] += 1;
NestWhile[(
(*If we've evaluated the function at least once already,
and we're past the 15000th evaluation (skipping ahead because
it starts out kind of uneventful), pause for 0.1 second for
visualization purposes, so we can "see" the avalanche play out.*)
If[p == 0 && m > 15000,
Pause[.1],
(*Indicate that after this point,
the function has been evaluated once this iteration by
"flipping p to 0"*)
p = 0];
(*trigger avalanche on the set of over-capacity points,
starting with just the set containing the newly-incremented point,
then aggregate the resulting list of lists of "hot" points into one
list with no duplicates*)
Union @@ (avalanche /@ #)) &, {{x, y}}, (# != {} &)];
m++;,{n, nmax}];
(*Output the final arrayplot from Monitor at the end*)
ArrayPlot[sandpile, PlotLabel -> "Iteration " <> ToString[m],
ImageSize -> Medium,
ColorFunction -> (If[# <= 4, GrayLevel[#/4], Magenta] &),
ColorFunctionScaling -> False],
ArrayPlot[sandpile, PlotLabel -> "Iteration " <> ToString[m],
ImageSize -> Medium,
(*Color all values by graylevel between 0 and four (0 - black, 4 - white)
and color over-capacity values in magenta.*)
ColorFunction -> (If[# <= 4, GrayLevel[#/4], Magenta] &),
ColorFunctionScaling -> False]]
P.S. I should be sleeping, but I made a gif of it... :)
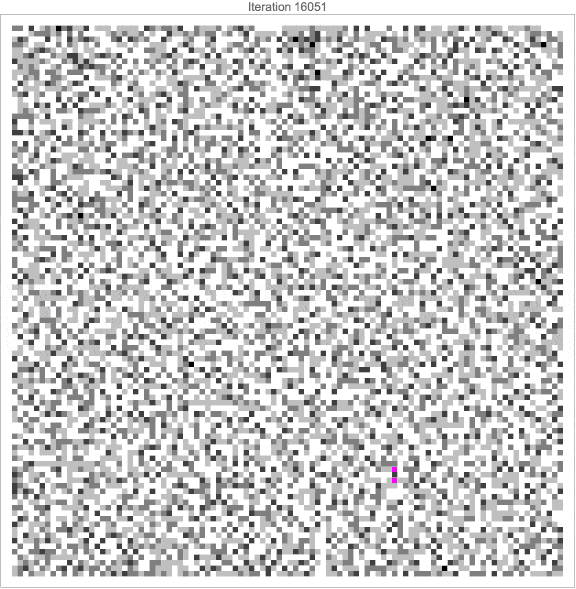
/@
in certain spots instead of setting up all theseDo
s! One advantage that has is that it's amenable to parallelization! $\endgroup$pile
essentially is depth-first: as it goes through the neighbors of{x,y}
, it calculates all the consequences of the first over-capacity neighbor it encounters before moving on to the next neighbor. Instead, it might help to essentially do it breadth-first: calculate the one-step effects of all the neighbors first, before calculating the knock-on effects of the cells the neighbors have affected. This might ease the recursion depth problem! $\endgroup$